Breakthrough AI Method WHAM: Accurately and Effectively Predicting 3D Human Motion in Videos
In the latest research, researchers from Carnegie Mellon University (CMU) and the Max Planck Institute for Intelligent Systems have jointly released an innovative AI method called WHAM (World ground Humans with Accurate Motion). This method has achieved a breakthrough in accuracy and efficiency in accurately estimating 3D human motion from videos.
3D human motion reconstruction is a complex process that involves accurately capturing and modeling the motion of the human body in three-dimensional space. When dealing with videos captured by mobile cameras in real-world environments, this task becomes even more challenging as these videos often contain issues such as foot slippage. However, researchers from CMU and Max Planck Institute for Intelligent Systems have successfully addressed these challenges through the WHAM method, achieving precise 3D human motion reconstruction.
There are two methods for restoring 3D human pose and shape from images: model free and model-based. It emphasizes the use of deep learning techniques in model-based methods to estimate the parameters of statistical body models. The existing video based 3D human pose estimation methods introduce temporal information through various neural network architectures. Some methods use additional sensors, such as inertial sensors, but they may cause interference. WHAM stands out by effectively combining 3D human motion and video context, utilizing prior knowledge, and accurately reconstructing 3D human activity in a global coordinate system.
This study addresses the challenge of accurately estimating 3D human pose and shape from monocular videos, emphasizing global coordinate consistency, computational efficiency, and realistic foot to ground contact. By utilizing the AMASS motion capture and video dataset, WHAM combines a motion encoder decoder network to convert 2D keypoints into 3D poses, with a feature integrator for temporal cues and a trajectory refinement network considering ground contact for global motion estimation, improving accuracy on non planar surfaces.
WHAM uses a unidirectional RNN for online inference and accurate 3D motion reconstruction, with a motion encoder for context extraction and a motion decoder for SMPL parameters, camera translation, and foot ground contact probability. The use of bounding box normalization techniques is helpful for extracting motion context. The pre trained image encoder for human grid restoration captures and integrates image features and motion features through a feature integrator network. The trajectory decoder predicts the global direction, while the refinement process minimizes foot slip. Training on synthetic AMASS data, WHAM outperforms existing methods in evaluation.
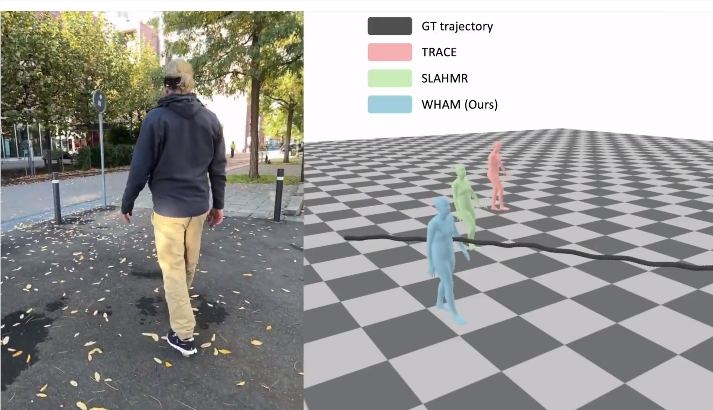
WHAM surpasses current state-of-the-art methods and demonstrates outstanding accuracy in frame by frame and video based 3D human pose and shape estimation. Accurate global trajectory estimation has been achieved by utilizing motion context and foot ground contact information, minimizing foot slip and improving international coordination. This method integrates the features of 2D keypoints and pixels, improving the accuracy of 3D human motion reconstruction. In field benchmark tests, WHAM demonstrated excellent performance in metrics such as MPJPE, PA-MPJPE, and PVE. Trajectory refinement technology further enhances global trajectory estimation and demonstrates the effectiveness of reducing foot slip through improved error metrics.
Overall, the main points of this study can be summarized as follows:
1. WHAM has introduced a groundbreaking approach that combines 3D human motion with video backgrounds.
2. This technology enhances the regression of 3D human pose and shape.
3. This method utilizes a global trajectory estimation framework that includes motion context and foot to ground contact.
4. This method solves the problem of foot sliding and ensures accurate tracking of 3D motion on non planar surfaces.
5. The WHAM method performs well on diverse benchmark datasets including 3DPW, RICH, and EMDB.
6. This method achieves efficient human pose and shape estimation in the global coordinate system.
7. The feature integration and trajectory refinement of this method significantly improve the accuracy of motion and global trajectory.
Through in-depth analysis and research, the accuracy of this method has been verified.
Paper URL: https://arxiv.org/abs/2312.07531
Project website: https://wham.is.tue.mpg.de/